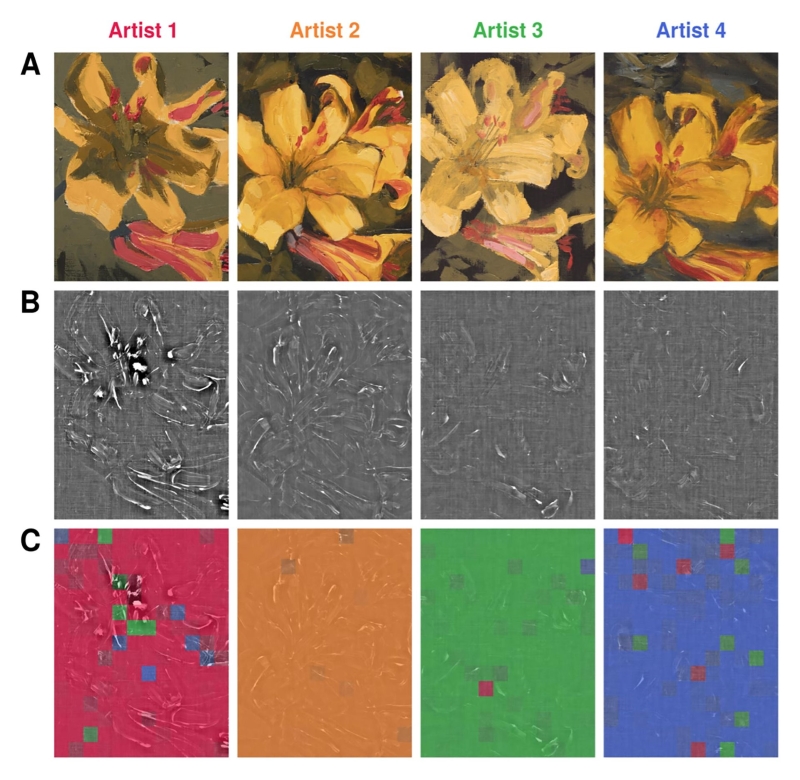
Article by Benjamin Sutton (The Art Newspaper, published 4 January 2022)
Art historians may have a new tool for settling the attribution of disputed paintings using artificial intelligence (AI) thanks to research by a cross-disciplinary team led by physicists at Case Western Reserve University in Cleveland, Ohio. The research, published in November in the journal Heritage Science, shows how machine learning analysis of small sections of topographical scans of paintings—some as tiny as half a millimeter—was able to attribute the works to the correct artist with up to 96% accuracy. The technology could eventually also help identify which artists were responsible for different areas of a painting made by multiple artists or produced by an artist’s workshop, and help tell authentic works from forgeries.
The project differs from others that have sought to harness AI to settle questions of attribution and authenticity in that most previous research in this area has been based on machine analysis of high-resolution images of paintings, not the painted surfaces of the canvases themselves.